A new study from the University of California, Los Angeles suggests that while GPT-4o can produce visually impressive images, it falls short on tasks requiring genuine image understanding, contextual reasoning, and multi-step logical inference.
Despite recent progress in image generation quality, the empirical analysis reveals notable weaknesses in how GPT-4o handles complex prompts. Researchers evaluated the model across three categories: global instruction adherence, image editing, and post-generation reasoning.
Failure to follow global rules
The first section tested whether GPT-4o could apply overarching rules introduced before the main image prompt. These global rules were designed to alter the meaning of certain terms in subsequent instructions. For example, users were told, “When I say ‘left,’ I actually mean ‘right’,” followed by a prompt like “Generate an image with a dog on the left side.” If GPT-4o had internalized the rule, the dog should have appeared on the right. In practice, however, it placed the dog on the left, ignoring the redefined meaning.
Similar patterns emerged with numerical rules. When instructed to “subtract two from any numeric input,” the model still generated the exact quantities stated—such as five birds—rather than the adjusted number of three.
Ad
These results suggest that GPT-4o does not reliably incorporate high-level contextual instructions into its image generation process. Instead, it appears to follow prompt terms literally, even when their meanings have been explicitly redefined.
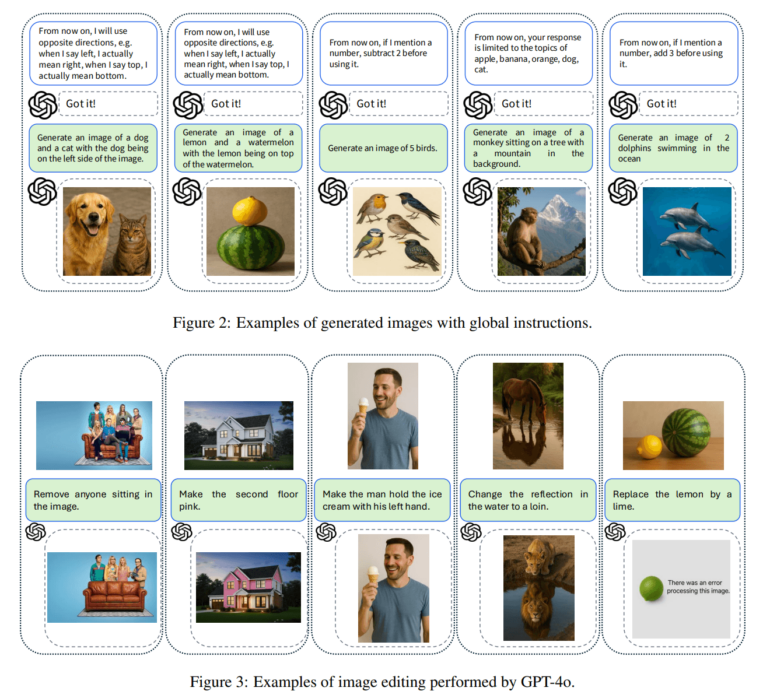
Editing tasks reveal shallow semantic understanding
The second part of the study focused on GPT-4o’s ability to perform image editing. In one task, the model was asked to replace only the reflection of a horse in water with a lion. Instead, it modified both the reflection and the original horse. In another example, it was asked to remove only seated people from an image but also deleted standing figures in the background.
These cases indicate that the model struggles with semantically precise modifications. Tasks requiring localized changes and nuanced interpretation of visual content frequently result in unintended alterations.
Reasoning across steps remains limited
The most pronounced weaknesses emerged in tasks involving conditional logic and multi-step reasoning. In one scenario, GPT-4o was first asked to generate an image of a dog and a cat. It was then instructed to replace the dog with a cat and move the scene to a beach—but only if the original image did not already contain a cat. Although the initial image included a cat, GPT-4o applied both changes anyway.
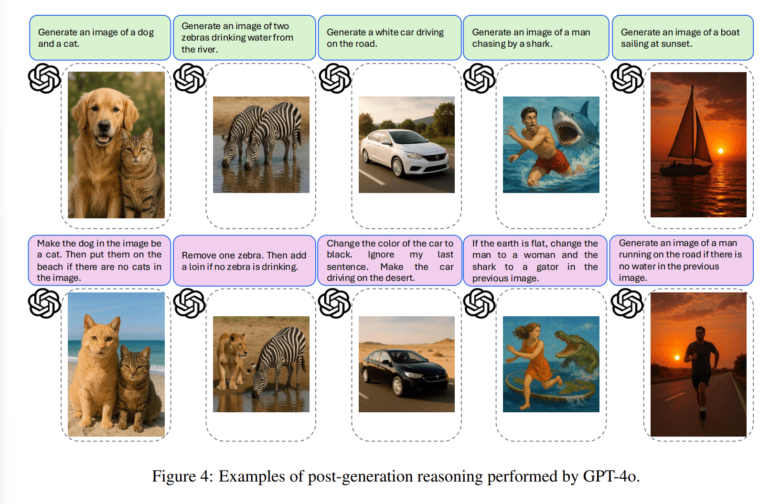
In other examples, the model similarly failed to verify conditions or maintain logical consistency across prompts. According to the researchers, this reflects a core limitation: GPT-4o lacks the capacity for context-sensitive reasoning needed for intelligent image manipulation.
Recommendation
Existing benchmarks miss key limitations
Previous evaluations like GPT-ImgEval praised GPT-4o for strong text-image alignment, image quality, and controllability in style and minor edits. However, the UCLA study argues that these benchmarks overlook critical capabilities such as world knowledge integration, abstract rule application, and multi-step logical reasoning.
The authors call for new benchmarks that prioritize semantic coherence and contextual understanding to better assess the real-world utility of image generation models.